A newly released paper, “Occupational Heterogeneity in Exposure to Generative AI” seeks to answer this question by assessing which occupations are most exposed to advances in AI language modelling and image generation capabilities.
The methodology involves calculating a standardised exposure score for each occupation based on its susceptibility to advances in generative AI. This score is determined using data on tasks and skills required for each occupation and information on the current state of AI technology. Exposure scores are standardised with a mean of zero and a standard deviation of one.
Additionally, the authors use regression analysis to investigate the relationship between different characteristics of occupations, such as education or wage level, and exposure to generative AI.
The study reveals that highly-educated, well-paid, white-collar occupations may be most vulnerable to these advances since these jobs often entail tasks that can be automated by generative AI, like writing reports or generating visual content. In contrast, jobs requiring more manual labour or interpersonal skills may be less impacted.
PR Specialists rank at number 34, Proofreaders at 54, Fundraisers at 62, and PR managers at 67. Interestingly, Chief Executives appear at 92, which seems improbable…
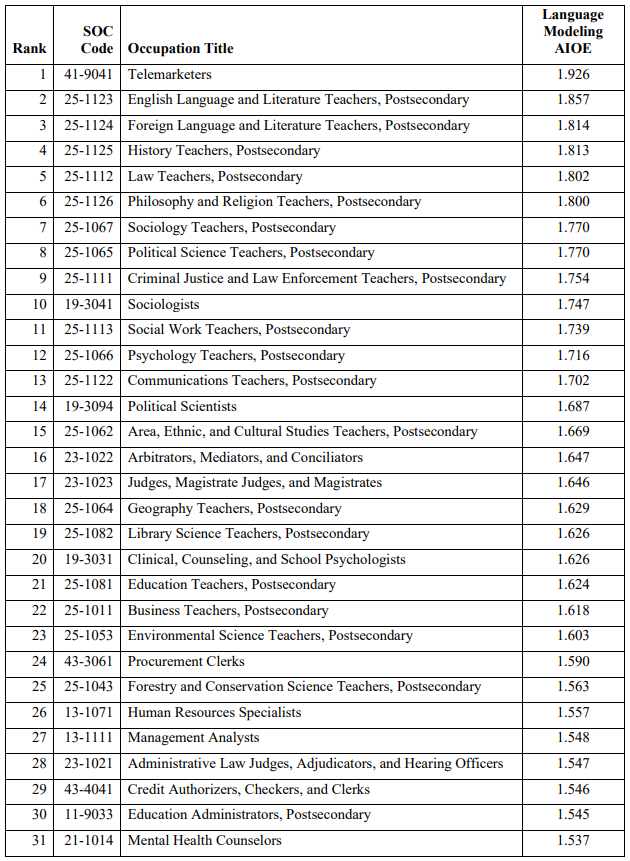
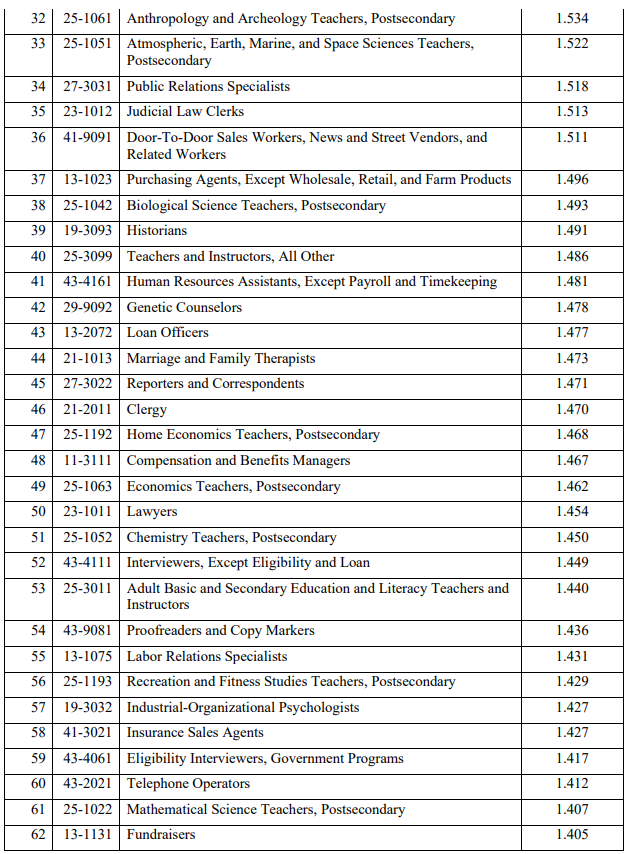
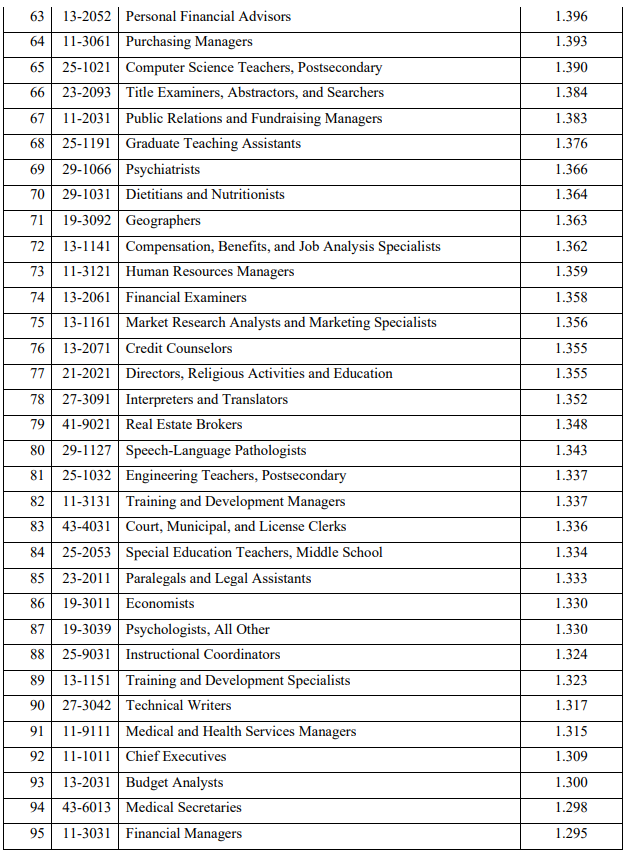
On the other end of the spectrum, with the least exposure, are Dancers at 774, Fitness trainers at 773, and Orderlies at 772.
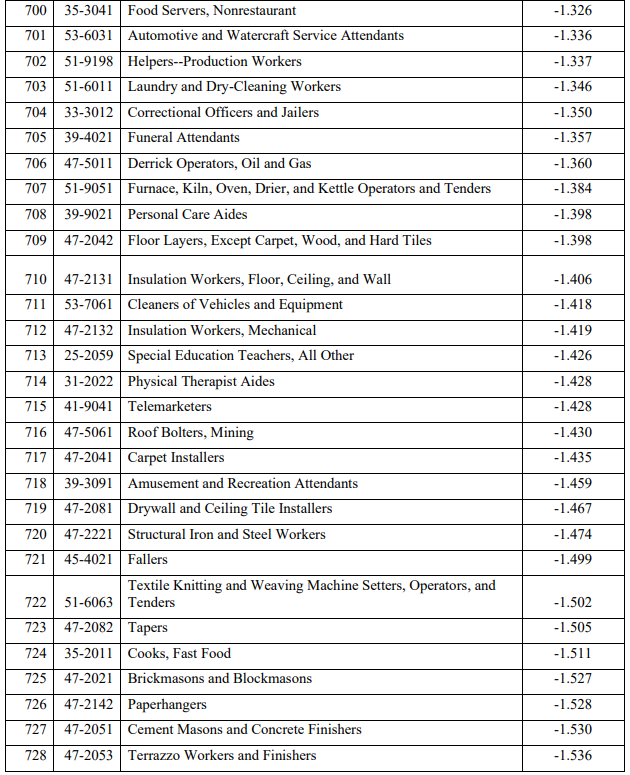
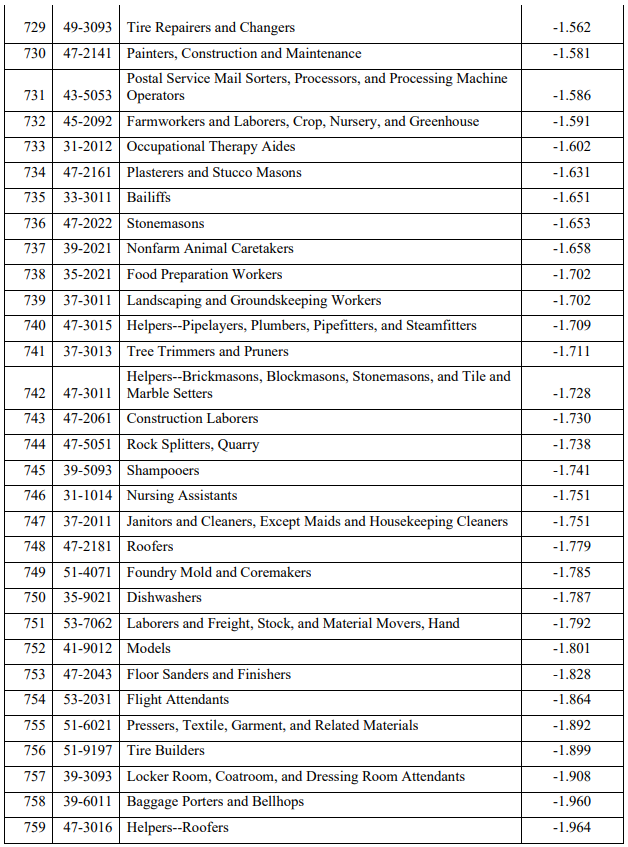
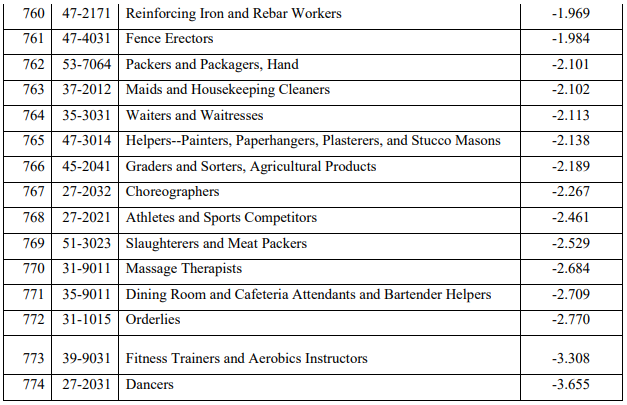
The paper also explores demographic variation concerning who will be most affected by generative AI. For instance, women and minorities may be disproportionately impacted if their jobs involve tasks more easily automated by generative AI.
As significant disruption looms, policy interventions may be necessary to help individuals adapt to the new demands of the labour market created by generative AI. This could include retraining programs for workers at risk of automation and policies promoting investment in new industries and technologies.
Felten, Edward W., Raj, Manav, and Seamans, Robert, Occupational Heterogeneity in Exposure to Generative AI (April 10, 2023). Available at SSRN: https://ssrn.com/abstract=4414065 or http://dx.doi.org/10.2139/ssrn.4414065